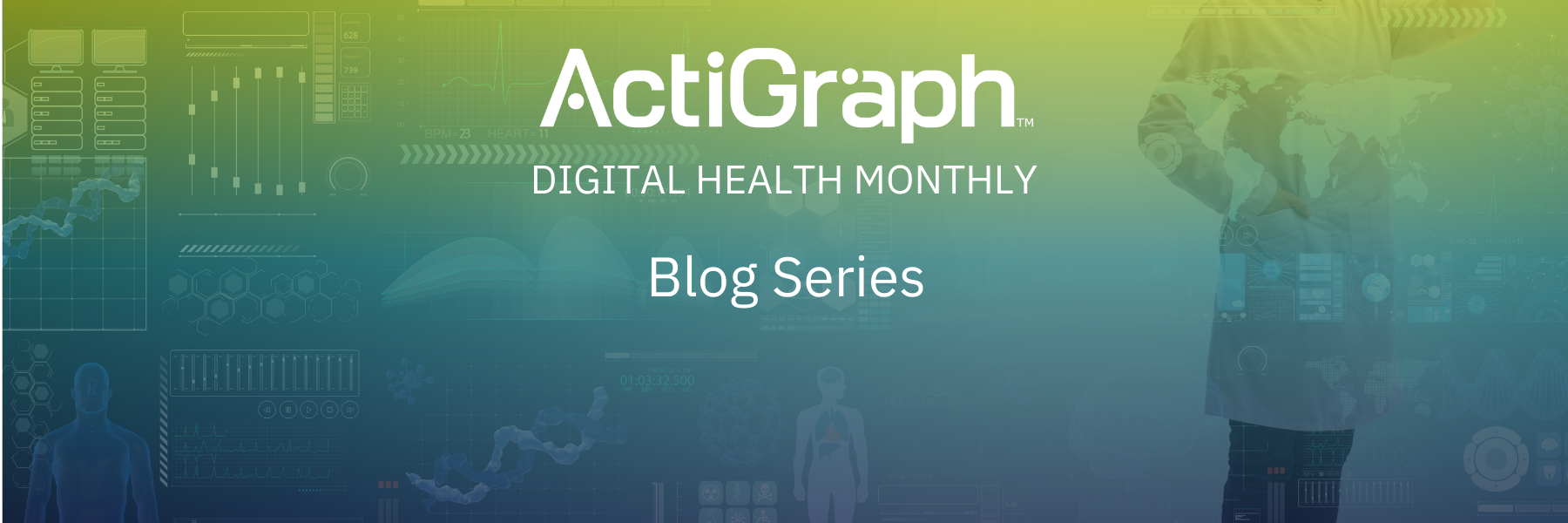
Wearable DHT 101: Foundations for Use in Regulated Clinical Trials
![]() |
Written by Christine Guo, PhD |
Innovation is exciting, but it can also come with challenges; innovation in the life sciences field, particularly clinical trials, is no exception. As technology advances and regulations evolve, working together to align on shared terminology and best practices can help us deploy innovations and realize their potential. This opportunity for shared dialogue was one of our main motivations behind starting the Digital Health Monthly webinar series, and so we decided to use our June 2024 session to review key considerations for deploying digital health technology (DHT) in clinical research. We hope that sharing some of our experiences in this regard will contribute to the effective utilization of DHTs in clinical trials to help deliver more effective treatments to patients safely and faster.
> Download our full eBook: Wearable DHT 101 for Clinical Research
The category of DHT can support a wide variety of public health and clinical use cases, but our focus here will be on wearable DHTs and the data derived from them as endpoints in the context of regulated clinical trials. As an introduction to this subject, I wanted to connect the dots from technical specification to regulatory acceptance with the goal of giving a holistic view to clinical trialists of this journey of research and evidence generation that includes DHTs.
In this blog post, we’ll expand on the following topics related to DHTs -
-
- Benefits of Continuous Sensor Data
- Scope of Wearable Use in Clinical Trials
- Wearable DHT Sensors and Measures
What is DHT?
Digital Health Technology (DHT) is a term formalized by the FDA as “a system that uses computing platforms, connectivity, software, and/or sensors, for health care and related uses” in the FDA’s recent guidance for DHT use for remote data acquisition in clinical investigations. DHTs can include tools that either remotely capture continuous sensor data (such as wearables, continuous glucose monitors, or an ECG patch) or tools that remotely capture discreet input (such as ePRO and eDiary). Typically, discreet input is simpler to process. There are some unique challenges that come with continuous sensor data, and we will focus more on that type of data from sensor-based, medical-grade wearable DHTs today.
Benefits of Continuous Sensor Data
Why do we bother with continuous sensor data if it's so complex? This type of data enables patient-centric digital transformation of clinical trials. With DHTs we can -
- Collect remote data from patient homes, reducing the barrier for participation in clinical trials
- Obtain objective measures of function, reducing biases due to culture differences or cognitive abilities
- Capture function in real life, revealing meaningful insights that are not visible or detected with traditional tools
The benefits of DHT-derived data in clinical trials are also recognized by the FDA; similar points were shared by an FDA colleague during a joint FDA and FNIH public workshop that I attended the day before this webinar, including -
- Richer data instead of snapshots
- Ability to detect rare events
- Data from patients who can otherwise not report
- New types of measurement
- Decentralized clinical trial measures reflecting real-world performance of drugs
Scope of Wearable DHT Use in Clinical Trials
Now we can put the continuous sensor data collected from wearable DHTs into context of their broader scope of use. The digital device itself is used to collect information about a Health Concept, such as function, behavior, and/or physiology. We can use these digital measures to generate either a clinical outcome assessment (COA) or a biomarker, and they can be used in a few different use cases such as endpoints to determine safety and efficacy, to improve clinical trial enrollment, for patient population enrichment, for dosing selection, or for monitoring adverse events.
In this blog, the focus is on the use of wearable data for supporting efficacy endpoints. Next, we walk through a concrete example of a commonly used measure, step count, to illustrate the journey of getting from sensor data to a clinical trial efficacy endpoint.
We start with a 3-axis accelerometer Sensor to capture acceleration data. In this case, the data collection is passive, so the User Input is simply to ask the participant to wear the device on their wrist, allowing us to collect the Raw Data of acceleration reflecting the movement of the wrist. The raw data is very complex and not easily interpretable, and so the next step is to use an Algorithm to derive a Feature, such as the detection of walking. This is something we can relate to the patient’s function, but this is still not something we can use as a measure. Since this data is variable within an isolated measurement period, we typically will use an Aggregation step to look at a reliable and well-defined Measure of mean walking bout duration over a 2-week period. Then we look at the Context of Use, we can use this digital measure as a Clinical Endpoint COA of mobility in a trial investigating a treatment for heart failure, for example. Hopefully this is a helpful illustration of the steps we need to think about when using sensor data to derive an efficacy endpoint.
Wearable DHT Sensors and Measures
An accelerometer is the most commonly used wearable DHT sensor, but other common sensors include gyroscope (rotational movement), microphone (speech or coughing detection), multiwavelength PPG (detecting vital signs like heart rate), barometer (altitude of device for fall detection), skin temperature, GPS, and touch screen (for participant input).
There is a variety of data that can be passively collected with wearable DHTs and used to derive digital measures of physical activity, gait and balance, sleep, vital signs, and cardinal symptoms of certain diseases:
I hope this discussion demonstrates that there is very rich information and numerous measurements that can be derived from even just a single medical-grade wearable device, and that you now have a better understanding of the basics of wearable digital health technology and how sensor data is used in the context of clinical trial endpoints.
Audience Q&A
Q: How is the ActiGraph device different from a consumer device?
A: Our device is made specifically to be fit-for-purpose for use in clinical trials. One major difference when using a medical-grade wearable is that the raw data is available, and we prioritize version control so the algorithm used to extract features from the raw data is not going to change over the course of a multi-year clinical trial, whereas many consumer device companies have proprietary algorithms that they can update at any time.
Q: Does Actigraph data provided classify as Features in your diagram? Do you support development of Measures?
A: It's a mix. As you can see in the diagram, there are several steps in deriving features and aggregation of measures. Most of the time we provide measures that are aggregated on the daily or some epoch level. For example, we talk about identifying a step, but a lot of times the aggregation of the step data needs to be tailored to a particular study to obtain a clinically meaningful measure for that clinical population, whether it’s a slowly progressing disease or a trial assessing a faster, more acute change.
Q: Has DHT data been used in registration trials?
A: Yes, the slide below shares multiple examples of DHT data used as primary or secondary endpoints in registered clinical trials.
Watch the full Digital Health Monthly webinar, DHT 101 for Clinical Research: Foundations, Regulations, and Real-World Applications, for more details about wearable use cases in regulated clinical trials as well as a deep dive into key considerations for evidence generation and operational deployment.