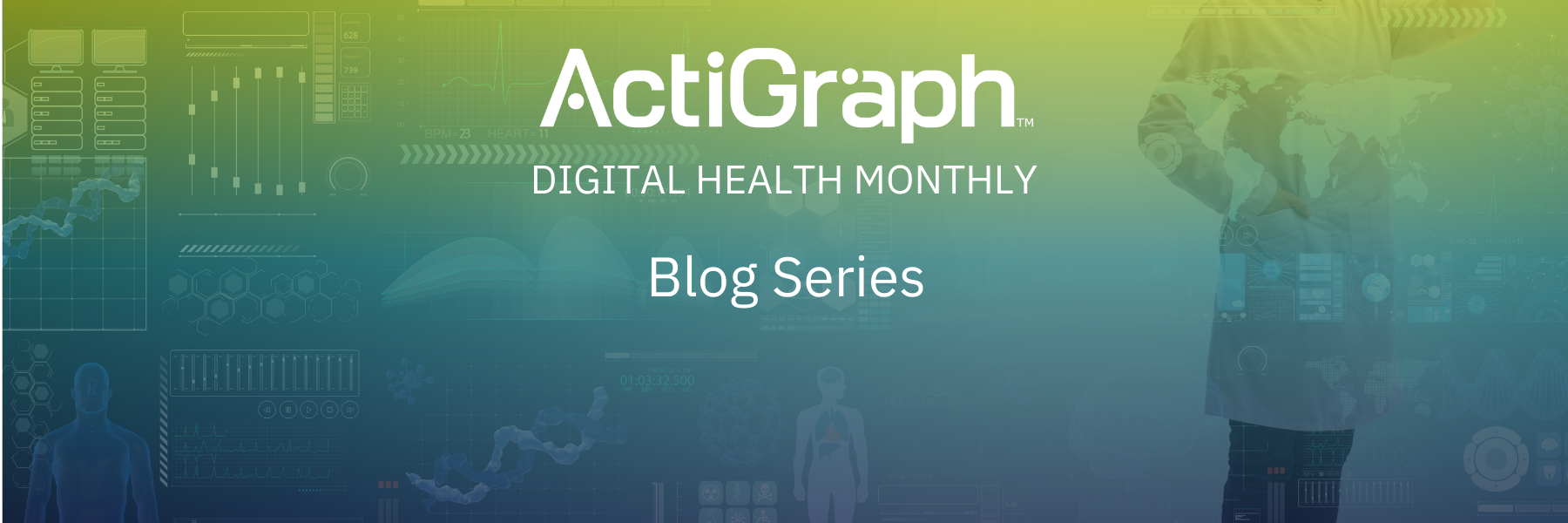
Filling the Sleep Health Gap: Advancing Patient-Centric Research with DHTs
![]() |
Written by Lindsey Snyder, PhD |
We know that sleep quality plays a major role in our health, recognized as a central pillar of population health along with nutrition and exercise (AASM, CDC). Unfortunately, millions of people each year suffer from sleep disorders, and sleep problems are common and are well known to affect the quality of life of patients in many chronic conditions. However, accurate, reliable and longitudinal measures of sleep can be somewhat of a challenge to collect using standard tools, such as polysomnography (PSG) and sleep diaries, each of which have known limitations.
Wearable digital health technologies (DHTs) have been used for decades by researchers to collect passive, objective, and continuous data to learn more about participants' sleep habits over time and in their home environment. This type of data can help advance our understanding of sleep health. This is particularly true in clinical research and drug development, where fit-for-purpose tools can provide reliable and accurate data about patient functioning and quality of life and help assess the effectiveness of new treatments. Highlighting this idea, the Digital Medicine Society’s DATAcc initiative recently launched the Core Digital Measures of Sleep resources.
How can advancements in DHTs help us fill the gap in addressing sleep health? In the second installment of our Digital Health Monthly scientific webinar series, we learned more about the practical considerations for collecting digital measures of sleep and review the latest capabilities for collecting data from wearable DHTs to inform sleep staging measures.
Sleep structure, significance, and tools for measurement
Even as late as the 1950s, sleep was thought of as a passive activity where the brain and body were inactive. However, we now understand that sleep is an active process necessary for sustaining quality of life even though we are at rest. Our first guest, Maxime Elbaz, PhD, Sleep Specialist and Chief Scientific Officer at BioSerenity, described the stages of the sleep, and the approximate distribution for normal adults sleeping 7-8 hours each night -
- (Non-REM) Light Sleep: 55%
- (Non-REM) Deep Sleep: 25%
- REM Sleep: 20%
However, disturbed sleep is quite common, affecting 1 in 3 people in France and Europe. In the short and medium-term, sleep disturbances can decrease productivity, reduce attention, and increase the risk of accidents and conflicts. In the long-term, they can lead to co-morbidities such as cardiovascular disease, depression, obesity, and diabetes. Therefore, including measures on sleep that can track disturbances and sleep patterns over time and in a natural environment are important to assess both short and long-term impacts on health.
While lab-based polysomnography (PSG) tests offer high precision when it comes to measuring sleep and sleep stages, connected devices for at-home capture of sleep data over days, months, or even years are available. These include medical grade wrist-worn wearables, portable EMGs, connected mattresses and pillows, and consumer-grade smartwatches and apps. There are multiple factors to consider when choosing the right DHT for your study, including validation of sleep measures, data integrity, data management and security, as well as aspects of usability such as ergonomics, wear tolerance, and required software.
While consumer-grade wearable devices can offer strengths such as improved consumer access and scalability, it is important to keep in mind the potential limitations such as automatic firmware updates, proprietary software and data processing (i.e., “black boxes”) that can limit data transparency and interpretation of results.
Technical advancements for sleep measurements using fit-for-purpose wearable DHT
Wearable DHTs that are fit-for-purpose for clinical research have been used since the 1980s to provide an understanding of how patients are sleeping in the real world. These devices use data from accelerometer technology to generate sleep and wake classification. Technical advancements such as multi-axis sensing, increased data storage that allows for raw data to be saved (not just epoch data), data transfer to the cloud and increases in battery life have made this technology easier to integrate in clinical research over the years.
Matthew Patterson, Senior Data Scientist at ActiGraph, showed sample wearable accelerometer data to demonstrate the sleep and wake classification from this type of data, which provides sleep measures of -
- total sleep time,
- wake after sleep onset (WASO),
- sleep efficiency, and
- fragmentation indices.
New sensor-based, wearable DHTs that utilize photoplethysmography (PPG) can detect blood volume changes in the microvascular bed of tissue, and this data is incorporated into models to generate measures of sleep architecture such as -
- REM time
- Light sleep time
- Deep sleep time
- Heart rate during sleep period
- Heart rate variability during sleep period
This type of data adds a higher resolution to help understand patients’ sleep characteristics and quality. Using raw PPG data collected from wrist-worn wearables, like the ActiGraph LEAPTM, Matthew and the data science team have been working on validating a model called SleepPPGNet. The model was developed using 4 different data sets; three of which are open-source data sets from sleepdata.org, and one internal data source. For pre-training and testing, ECG and finger-based PPG data sets were used, training was done on the MESA PPG data set and testing of the model was performed using the internal wrist-based PPG data set.
When tested, the performance of sleep stage classification of SleepPPGNet was similar to levels seen in interrater reliability on PSG tests, and therefore the team is optimistic that the model will perform well in the field.
Wearable-based sleep measures, and these new opportunities to improve the accuracy and resolution of sleep assessments with multisensor wearables, creates a scientifically validated and scalable solution for researchers to incorporate patient-centric sleep assessments. While there are unique considerations for choosing the right sleep assessment for a particular study, digital measures of sleep with fit-for-purpose wearable DHTs can help fill the gap in sleep assessments in clinical research.
Audience Q&A
Q: How does the accuracy of the sleep detection algorithm that uses PPG compare to the sleep detection algorithm that only uses accelerometry (not the PPG signal)?
A: Accuracy of ActiGraph’s DACNN model to predict sleep / wake using accelerometer alone is 80.1, whereas the median accuracy of the PPGSleepNet model is 78.1. However, the PPGSleepNet model predicts 4 stages of sleep (wake, REM, light and deep sleep).
Q: As with finger oximetry, do you find variations of measurements with wrist actigraphy based on an individual’s skin color?
A: Data was collected using the PPG sensor on the ActiGraph LEAP to generate heart rate measures and was tested on all skin types (Fitzpatrick I to VI). The light intensity from the PPG sensor was adjusted based on skin type. In this testing, no significant difference in measurement accuracy was seen between skin types.
To learn more about the ActiGraph LEAP capabilities, you can watch the webinar, “LEAP into the Future of Patient-Centered Research: Introducing the Latest Innovation in Wearable Technology.”
If you're interested to learn more about how digital sleep measures can help assess treatment effectiveness and patient functioning in clinical trials, read our white paper, “Opportunities to Close the Gap in our Understanding of Sleep Health with Fit-for-Purpose Wearable Digital Health Technologies.”