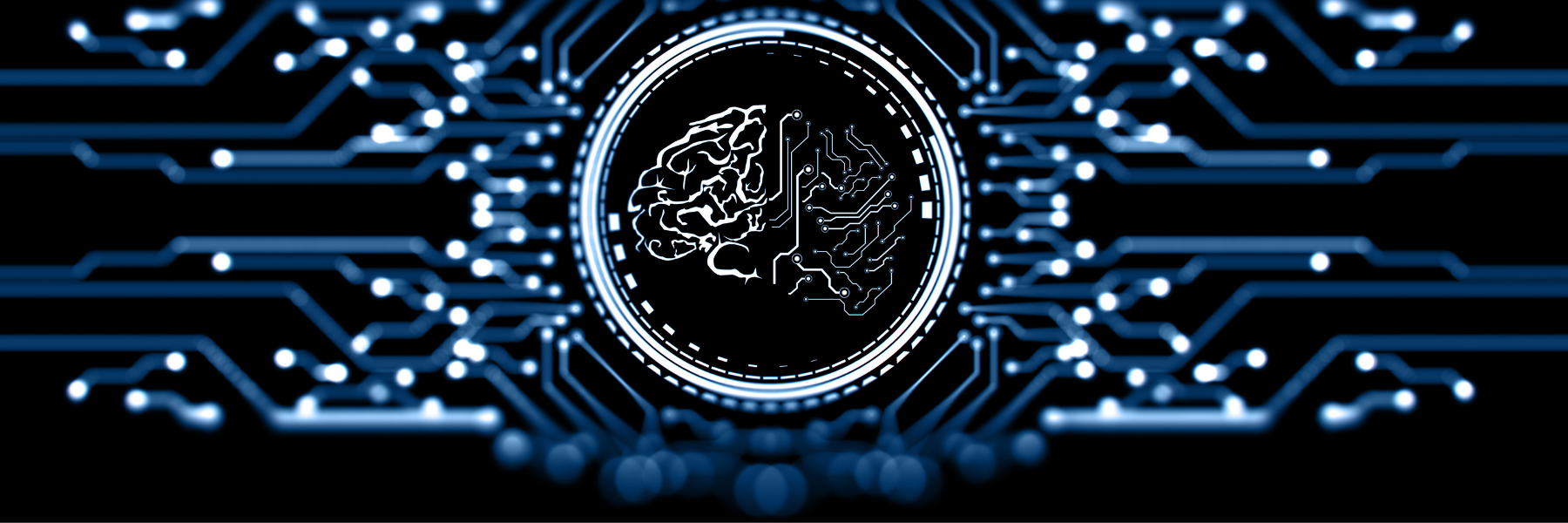
Demystifying AI: A Review of the Fundamentals and Applications in Drug Development
![]() |
Written by Ali Neishabouri, PhD |
Artificial Intelligence (AI) has recently become a household term, with its influence permeating many aspects of life, from the rise of large language models like ChatGPT to the advent of self-driving cars and AI-generated art. Companies across many industries are exploring the use cases and potential benefits that AI-based tools can bring to their operations, prompting regulatory bodies to assess the implications. The field of drug development is no exception. However, to harness the full potential of these tools, it is essential to grasp the fundamental concepts and understand how to apply AI effectively in specific contexts. In this blog post, we'll explore the history of AI, clarify key concepts and common questions, and delve into its use in drug development.
Is AI a recent concept?
The quest for a machine capable of emulating human behavior is not recent. Mechanical Turks (automated chess players), the differential engine, electronic calculators, and computers have been successively proposed to try and replicate intelligence, even though intelligence itself is a rather nebulously defined concept. Advances in each aspect of artificial intelligence, e.g., calculation, typically led to another aspect, e.g., creativity, being hailed as the new definition for what distinguishes human intelligence.
However, advances in the early to mid-20th century led some to predict that the goal of replicating human intelligence was within reach. For example, Alan Turing famously proposed a test for evaluating whether this goal had been attained, which consists of a conversation between a human and an artificial agent. After the conversation, the human is tasked to determine whether he/she is talking with another human or an artificial intelligence.
The term "artificial intelligence" (AI) was coined by John McCarthy, an American computer scientist and cognitive scientist, in 1956. He used the term to describe the field of study that aims to create machines capable of intelligent behavior. McCarthy is often seen as one of the founding figures of AI and played a significant role in shaping the field's direction through his work and contributions.
Machine Learning (ML) is a subset of Artificial Intelligence (AI) that focuses on the development of algorithms and statistical models that enable computers to perform tasks without explicit instructions. Instead of following specific commands, these systems learn from and make decisions based on data. While the original goal of machine learning models was the same, i.e., minimizing a cost function, the various approaches taken to reach this goal led to multiple and varied outcomes, such as image generation, text generation, image classification, and 3-d reconstruction.
Are AI models always changing?
While AI models can change, this is not always the case. AI models' development is typically comprised of two phases. First, during the training phase, a model is exposed to data and told to learn from it. Second, once a model is trained, it is typically used to predict or generate future data based on what it learned (this is called inference).
AI models are usually first developed in this order, but in practice, models can be trained/re-trained at any point. A large language model (LLM) can be "fine-tuned" after training, to improve its performance on a particular task. One benefit of this fine-tuning approach is that it allows the model to leverage as much data as possible as information becomes newly available. But this fine-tuning is not necessary for the application of AI, and the user is the one deciding whether to take this approach to change a model or not.
Are all Machine Learning models a “black box”?
The term black box is often used to describe a process where the inputs and the outputs are known, but the functions used to transform the input to the output are either not transparent or unknown.
When it comes to machine learning models, there is a continuum of how well the functions can be explained depending on the model. Some models are as simple as a linear fit to a curve (Linear regression, Ridge, Lasso, etc.). Some ML models, such as Random Forests, can be complex but have built-in mechanisms to report how they reach their decisions, and some can become so complex that it becomes very difficult to explain how they generated the outputs given their input.
Despite active research in this domain1, the most powerful models currently exceed our capacity to explain their results, just as we are incapable of explaining the exact steps a human brain takes to differentiate a photo of a cat from that of a dog. The lack of explainability does present challenges2 for using the most complex models. We can, however, measure the performance of the models and identify their limitations, which we can keep in mind as we interpret and apply them in given situations.
Are AI models always better than simple analytical models?
This is a slightly difficult question to answer as there is no definitive threshold for when a model becomes an AI model. All machine learning models rely on mathematical formulas to discover patterns and structures in the data presented to them. In general, the more complex a model is, the more capable it is of dealing with complex patterns. However, this capability comes at the cost of the model needing more data to learn and correctly recognize these complexities.
Also, it is very important to use high quality data to train any AI/ML model. If the source of the training data is incomplete, not validated, or unreliable, then the output of the model will be as well; a common phrase used to express this concept is “garbage in, garbage out.”
The task of learning patterns from data can and is also accomplished by humans, of course. We usually call these models heuristics, or a simple and efficient set of rules used to make decisions. In the absence of large amounts of high-quality data, and thanks to the human's domain knowledge, heuristic models can often show good performance and can even out-perform machine learning models.
How can AI support the use of sensor-based Digital Health Technologies (DHT), such as wearable devices?
Sensor-based DHTs leverage continuous data collected from the embedded sensors. Continuous raw sensor data has higher levels of complexity and dimensionality compared to questionnaire-based data used in conventional clinical outcome assessments. AI/ML techniques can provide powerful analytics to reveal patterns in the complex sensor dataset that are not possible to uncover using simpler methods.
One benefit of sensor-based DHTs is the large volume of data collected, particularly if the data is of high-quality. The more data available for training AI/ML models, the more this can improve the accuracy of the outcomes. As pointed out in the last section, complexity of the model doesn’t always mean that it is “better”, i.e., will produce more accurate outcomes (particularly if the data isn’t high-quality). However, the richness and volume of sensor data can be beneficial when more complex AI/ML models are needed to analyze and predict highly variable and complicated results, such as health outcomes, based on a variety of behavioral and physiological inputs.
Does the FDA accept the use of AI in clinical development?
The FDA has cleared and approved medical products leveraging AI/ML technologies and is playing an active role in guiding the responsible adoption of AI/ML in the drug development process3. The first AI-enabled medical device was approved by the FDA in 2015, and since 2022, FDA has published a full list of AI/ML-enabled medical devices that they have authorized4. In 2023, the 171 medical devices added to this list accounted for a ~30% increase year-over-year. This trend is expected to continue based on estimated funding growth in this area as well5.
The FDA recently used AI/ML models to identify a suitable patient population for a drug therapy. A team at the Center for Drug Evaluation and Research (CDER) used AI/ML algorithms to develop a clinical scoring rule that could identify patients that would most likely benefit from the drug anakinra (Kineret) for the treatment of COVID-196. This was the first time AI/ML models were approved for use in patient identification, but the authors of the Spotlight on CDER Science report suggest that similar approaches could be applied in patient selection for clinical trials7.
In addition to patient selection, AI can be applied used in clinical research to support drug submissions through help with site selection, to enhance adherence, in data collection (such as the use of wearables in clinical trials), analysis of real-world data (RWD), and in clinical endpoint assessment. The number of regulatory submissions to CDER with AI/ML components increased from one submission in 2016 to 132 submissions in 20218, illustrating the rapid growth in the use of these tools.
Artificial Intelligence is not a brand-new concept, but the widespread use of AI across a variety of industries and applications is growing. The ability of AI/ML models to identify complex patterns can help us solve difficult analytical challenges, but doing so effectively often requires large, high-quality data sets. This last concept is one that we appreciate at ActiGraph, because sensor-based DHTs capture data continuously over hours, days, weeks or longer, providing a large volume of data, and our team puts a lot of effort into the design and deployment of DHTs to maximize high-quality data collection. AI/ML algorithms are widely used to generate sensor-derived health outcomes for clinical research and development and have already been part of many regulatory submissions of drug and device products. With ethical and responsible use of AI, we expect these tools will becoming increasingly impactful in clinical tool development, adherence monitoring, patient recruitment/enrichment, advanced trial design and post-marketing surveillance.
References
1. Haoyan Luo and Lucia Specia, “From Understanding to Utilization: A Survey on Explainability for Large Language Models” (arXiv, February 21, 2024), https://doi.org/10.48550/arXiv.2401.12874.
2. Samuel Gehman et al., “RealToxicityPrompts: Evaluating Neural Toxic Degeneration in Language Models,” in Findings of the Association for Computational Linguistics: EMNLP 2020, ed. Trevor Cohn, Yulan He, and Yang Liu (Findings 2020, Online: Association for Computational Linguistics, 2020), 3356–69, https://doi.org/10.18653/v1/2020.findings-emnlp.301; Laura Weidinger et al., “Ethical and Social Risks of Harm from Language Models” (arXiv, December 8, 2021), https://doi.org/10.48550/arXiv.2112.04359.
3. U.S. Food and Drug Administration. FDA Releases Two Discussion Papers to Spur Conversation about Artificial Intelligence and Machine Learning in Drug Development and Manufacturing. https://www.fda.gov/news-events/fda-voices/fda-releases-two-discussion-papers-spur-conversation-about-artificial-intelligence-and-machine. Accessed 8/22/2024.
4. U.S. Food and Drug Administration. Artificial Intelligence and Machine Learning (AI/ML)-Enabled Medical Devices. https://www.fda.gov/medical-devices/software-medical-device-samd/artificial-intelligence-and-machine-learning-aiml-enabled-medical-devices. Accessed 8/22/2024.
5. McNabb N, Christensen E, Rula E, Coombs L, Dreyer K, Wald C, Treml C. Projected Growth in FDA-Approved Artificial Intelligence Products Given Venture Capital Funding. Journal of the American College of Radiology (2024), 617-623, 21(4), https://doi.org/10.1016/j.jacr.2023.08.030.
6. Liu, Q. et al. Using Machine Learning to Determine a Suitable Patient Population for Anakinra for the Treatment of COVID‐19 Under the Emergency Use Authorization. Clin Pharmacol Ther 115, 890–895 (2024), https://doi.org/10.1002/cpt.3191.
7. U.S. Food and Drug Administration. Regulatory Education for Industry (REdI) Annual Conference 2024: Innovation in Medical Product Development. https://www.fda.gov/drugs/news-events-human-drugs/regulatory-education-industry-redi-annual-conference-2024-innovation-medical-product-development. Accessed 8/22/2024.
8. Liu, Q. et al. Landscape Analysis of the Application of Artificial Intelligence and Machine Learning in Regulatory Submissions for Drug Development From 2016 to 2021. Clin Pharmacol Ther 113, 771–774 (2023), https://doi.org/10.1002/cpt.2668.