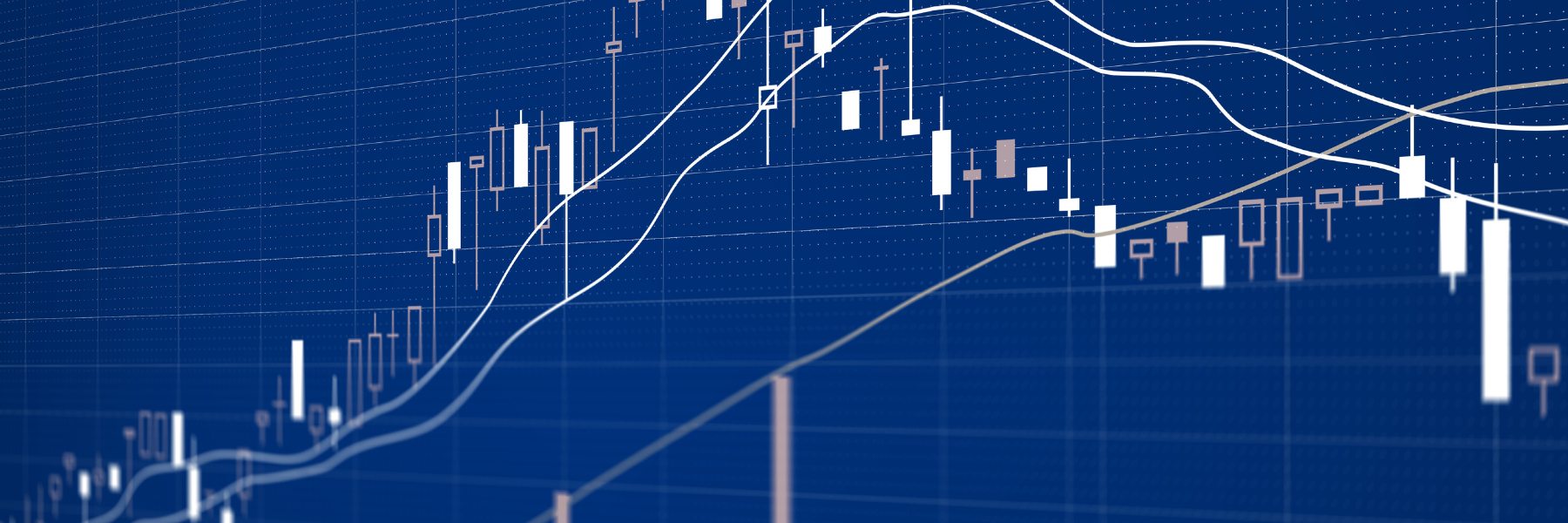
Case Study: Evaluating and Selecting Digital Measures in Large-Scale Population Studies
![]() |
Written by Andy Liu, PhD |
Sensor-based DHTs offer a new opportunity to collect data for novel outcome measures in public health research in an objective, non-invasive, and cost-effective manner. Researchers can use DHTs to collect a high volume of continuous data in the patient’s natural environment over an extended period. A diverse set of functional measures can be derived from wearable data, including physical activity, sleep, gait and walking characteristics.
While there are many advantages to using DHTs to collect data for population health research, there are also challenges that arise from the complex nature of this data. Given that there are unique considerations for deriving outcome measures from wearable digital health technology (DHT) data, how can a study team select a digital measure that best fits a particular study and context of use?
> Download our White Paper, Journey to the Right Digital Endpoint, to learn more about a framework to derive reliable clinical outcome scores in clinical trials.
There are a few questions that can be asked to help guide teams to the outcome measure with the best measurement properties:
- Which measure is most relevant to the concept of interest?
- What is the best data aggregation approach?
- How many days of data should we collect?
- How should missing DHT data be handled?
To address these questions, we developed a framework to evaluate DHT-derived measures based on the FDA’s recommendations of a well-defined and reliable outcome measure.
Digital Endpoint Evaluation Framework
When conducting the analysis with complete data:
- How relevant is the digital measure to a trait of interest? (i.e., how physically active a participant population is)
- What is the reliability of a digital measure from a day-to-day standpoint?
When conducting the analysis with incomplete data:
3. How reliable is the digital measure when data is missing?
4. How sensitive is the digital measure to bias when data is missing?
Case Study: Evaluating Physical Activity Measures for Diabetes Research
Using this framework, we leveraged a publicly available DHT dataset from the NHANES 2013-2014 cohort to determine which physical activity measure is most robust and appropriate to differentiate between groups of individuals with or without diabetes.
We supposed a scenario in which a study team has identified a list of DHT-derived physical activity (PA) measures and would like to determine which one(s) would be best suited as an outcome measure. In this case, we examined two cut point-based digital measures, MVPA and non-sedentary time, and two percentile-based digital measures, 80th percentile counts and 95th percentile counts.
- How relevant is each of the digital measures to our trait of interest in diabetes?
To answer this question, we analyzed whether each measure could differentiate between individuals with diabetes and individuals who don’t have diabetes. Each measure showed a statistically significant difference between control and diabetes groups (A, Figure 1). However, each of these measures is highly correlated with each other (the 95th percentile being slightly less correlated with the other measures) (B, Figure 1). This assessment shows that all these digital measures are relevant to reveal lower levels of physical activity in patients with diabetes. But based on this assessment alone, it’s still unclear which measure would be the best outcome measure since each performs at a similar level.
Figure 1.
2. What is the reliability of each of the digital measures?
To assess reliability, we first calculate the single day intra-class correlation coefficients (ICC) for each of the digital measures. Typically, an ICC value of 0.8 is used as a gold standard to determine if a measure can be considered reliable. None of the digital measures’ single day ICC reaches 0.8 (A, Figure 2), but we can improve the reliability of a measure by averaging across multiple days. By doing this calculation, we can estimate how many days are needed to reach an ICC of 0.8 or higher for each digital measure. When we do this calculation, we can see that each measure achieves an ICC of 0.8 or higher when we average just two days of data, with the 95th percentile activity measure performing best, requiring less data to achieve a reliable estimation (B, Figure 2).
Figure 2.
A Simulation Framework to Assess the Impact of Data Missingness
A common approach used to handle data missingness in studies using DHTs is to set a minimum wear time threshold, and then only include days that meet this requirement. Typically, studies will use a threshold for wear time such as 10, 16, or 20 hours as the minimum data requirement for it to be considered a valid day. To understand the effect of data missingness on each digital measure of PA, we developed a simulation framework.
In the simulation framework, we take empirical patterns of missingness from the NHANES data set and superimpose these patterns onto complete datasets from individuals with high adherence (<3% of data missing). In this way, we can simulate a data set including many individuals as if they only wore the device for 10, 16, or 20 hours per day, and have a robust data set to evaluate the effect of wear time (i.e., data missingness) on reliability and susceptibility to bias for each digital measure of PA.
3. How reliable is the digital measure when data is missing?
First, we look at the ICC for each measure as a function of wear time. We observed that a wear time of 10 hours per day greatly reduced the single day ICC values (A in Figure), hence requiring a much higher number of days of data to achieve a reliable ICC level (B in Figure). However, the reduction in reliability is much less when comparing the ICC values for 16 and 20 hours of wear time, requiring approximately two and a half to three days of data to reach a reliable level of ICC (0.8) for each of the digital measures of PA, with the 95th percentile requiring slightly less days of data.
Figure 3.
4. How sensitive is the digital measure to bias when data is missing?
Missing data can also cause bias in digital measures. For example, when we plot MVPA minutes versus minutes of wear time, there is a clear positive relationship between the two, with higher wear time correlating with more MVPA minutes. This suggests that the values of MVPA can be biased by how much a participant wears the device, i.e., data missingness.
Using the same simulation framework, we can evaluate the amounts of bias caused by data missingness for each digital measure. We computed a bias ratio, which is defined as:
(simulated_value – gold_standard_value) / gold_standard_value
Here, negative values indicate a measure will be underestimated when there is missing data, and positive values indicate a measure will be overestimated when there is missing data. Bias ratio values closer to zero mean that there is less bias, and values further from zero mean that there is more bias.
In our analysis, we observe that cut point-based measures of the amount of activity such as MVPA and non-sedentary time tend to have higher bias, especially when there is more missing data when using 10-hour wear time as a threshold. However, percentile-based measures such as the 80th and 95th percentile of activity counts are observed to have lower bias ratio, suggesting that these measures may be more robust to non-wear in the context of bias.
Figure 4.
Putting the Evaluation Framework Results Together
Now, we can bring all these results together into a table and systematically evaluate the strengths and weaknesses of each measurement to help us decide which of these measures might be best to use as a potential clinical endpoint in a diabetes study.
- Which measure is most relevant to our concept of interest?
Column A: In this example, all digital measures of PA can strongly differentiate between diabetic groups (higher values indicate a stronger association, with a value of 1.3 corresponding to a p-value of 0.05).
2. Which measure is most reliable?
Column B: The number of days needed to reach an ICC value of 0.8 gives us an idea of how many days of data would need to be collected to obtain a reliable estimate of the measure (fewer days indicate higher reliability).
3. Which measure’s reliability is least affected by data missingness?
Column C: We can look at this information to see the ICC as a function of wear time and calculate how many days of data would be needed to reach an ICC of 0.8 in each condition. This information helps us make an informed decision based on the adherence rates of the study and select a measure that minimizes the number of subjects excluded from the study.
4. Which measure is least prone to bias due to data missingness?
Column D: A bias ratio closer to zero means that there is less bias in the measure. It is important to consider how bias ratio may affect downstream analysis; for example, in a longitudinal analysis comparing MVPA at week 0 vs week 10, if compliance (i.e. wear time) decreases throughout the study, MVPA will be underestimated at a greater degree as the study progresses. This could lead to a conclusion that MVPA is decreasing over time, but in actuality it is wear time that was decreasing. However, if compliance was high throughout the study, MVPA may still be an appropriate measure.
Case Study Conclusions
This case study demonstrates several important points when it comes to selecting a digital measure as a study endpoint. First, different measures derived from the same data can have different statistical properties, and the minimum data requirement for the study depends on the measure, the study design, and the adherence rates in the study.
Additionally, reliability of the measure must be considered from both a complete data and incomplete data perspective, as the amount of data needed to reach an ICC of 0.8 changes based on wear time. Bias of a measure is also an important consideration and can be impacted by wear time.
For example, we saw that the MVPA measure has good reliability – the ICC was similar to the other PA measures, and 1.5-2 days of data was required to reach an ICC of 0.8. However, the bias ratio of MVPA increases as wear time decreases, meaning that data missingness has a greater impact on MVPA and could possibly lead to erroneous conclusions if device wear time adherence is low. While it may be possible to normalize MVPA values by daily wear time, this assumes an even distribution of MVPA throughout the day – an assumption that may not always be valid and can produce extreme values with low wear (data not shown).
In summary, when choosing a digital measure, it’s important to look at the adherence rates in conjunction with the statistical properties of the measure. The best option for a digital measure is one that maximizes reliability, minimizes bias, and excludes as few individuals as possible.
In this case study, the percentile-based measures of PA showed superior statistical properties, but they are less intuitive than MVPA and non-sedentary time.
- In a diabetes clinical trial, percentile-based measures might be a good option as they are relevant, reliable, and not very susceptible to bias.
- In contrast, it may be difficult to implement a public health recommendation by advising individuals with diabetes to increase their 80th percentile throughout the day instead of advising them to increase MVPA by 10 minutes per day.
Recommendation
The potential of wearable DHTs to enhance public health research requires the study team to apply the right statistical approach to process and analyze digital measures to avoid biases from data missingness and maximum the value of the rich digital data.
Visit our Population Health Research webpage to learn more about how ActiGraph can help you maximize the value that wearable data can add to your next cohort study.